The Paradox of Overfitting
I wrote my master's thesis at the Dutch National Research Institute for Mathematics and Informatics (CWI) in Amsterdam. The subject was the problem of overfitting in machine learning and how to prevent it.
Complexity theory and statistics are often considered very abstract
and difficult to study. A large part of my
work was dedicated to writing a comprehensible theoretical
introduction and to build a graphical tool that visualizes the
problems involved. Apart from that I also experimentally verified the
correctness of a novel approach to prevent overfitting: mixture MDL.
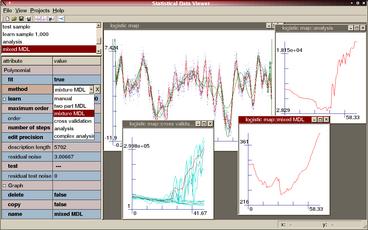
Screenshot of the Statistical Data Viewer
For an overview of my thesis read the summary. The thesis or parts of it can be downloaded below. Especially the theoretical part is interesting to students. It addresses a number of basic topics that are usually neglected in undergraduate courses. Among them are the different definitions of a good model, of overfitting and of a number of key mathematical concepts like Kolmogorov complexity, individual randomness and typicallity.